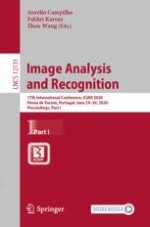
2020 | OriginalPaper | Buchkapitel
Exploring Workout Repetition Counting and Validation Through Deep Learning
verfasst von : Bruno Ferreira, Pedro M. Ferreira, Gil Pinheiro, Nelson Figueiredo, Filipe Carvalho, Paulo Menezes, Jorge Batista
Erschienen in: Image Analysis and Recognition
Aktivieren Sie unsere intelligente Suche, um passende Fachinhalte oder Patente zu finden.
Wählen Sie Textabschnitte aus um mit Künstlicher Intelligenz passenden Patente zu finden. powered by
Markieren Sie Textabschnitte, um KI-gestützt weitere passende Inhalte zu finden. powered by