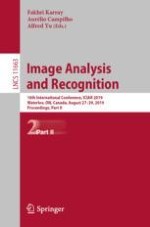
2019 | OriginalPaper | Buchkapitel
Foothill: A Quasiconvex Regularization for Edge Computing of Deep Neural Networks
verfasst von : Mouloud Belbahri, Eyyüb Sari, Sajad Darabi, Vahid Partovi Nia
Erschienen in: Image Analysis and Recognition
Aktivieren Sie unsere intelligente Suche, um passende Fachinhalte oder Patente zu finden.
Wählen Sie Textabschnitte aus um mit Künstlicher Intelligenz passenden Patente zu finden. powered by
Markieren Sie Textabschnitte, um KI-gestützt weitere passende Inhalte zu finden. powered by