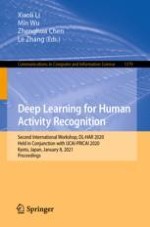
2021 | OriginalPaper | Buchkapitel
Human Activity Recognition Using Wearable Sensors: Review, Challenges, Evaluation Benchmark
verfasst von : Reem Abdel-Salam, Rana Mostafa, Mayada Hadhood
Erschienen in: Deep Learning for Human Activity Recognition
Verlag: Springer Singapore
Aktivieren Sie unsere intelligente Suche, um passende Fachinhalte oder Patente zu finden.
Wählen Sie Textabschnitte aus um mit Künstlicher Intelligenz passenden Patente zu finden. powered by
Markieren Sie Textabschnitte, um KI-gestützt weitere passende Inhalte zu finden. powered by