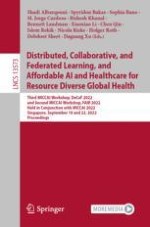
2022 | OriginalPaper | Buchkapitel
Incremental Learning Meets Transfer Learning: Application to Multi-site Prostate MRI Segmentation
verfasst von : Chenyu You, Jinlin Xiang, Kun Su, Xiaoran Zhang, Siyuan Dong, John Onofrey, Lawrence Staib, James S. Duncan
Erschienen in: Distributed, Collaborative, and Federated Learning, and Affordable AI and Healthcare for Resource Diverse Global Health
Verlag: Springer Nature Switzerland
Aktivieren Sie unsere intelligente Suche, um passende Fachinhalte oder Patente zu finden.
Wählen Sie Textabschnitte aus um mit Künstlicher Intelligenz passenden Patente zu finden. powered by
Markieren Sie Textabschnitte, um KI-gestützt weitere passende Inhalte zu finden. powered by