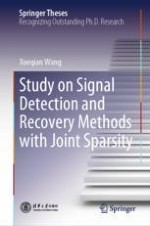
2024 | OriginalPaper | Buchkapitel
1. Introduction
verfasst von : Xueqian Wang
Erschienen in: Study on Signal Detection and Recovery Methods with Joint Sparsity
Verlag: Springer Nature Singapore
Aktivieren Sie unsere intelligente Suche, um passende Fachinhalte oder Patente zu finden.
Wählen Sie Textabschnitte aus um mit Künstlicher Intelligenz passenden Patente zu finden. powered by
Markieren Sie Textabschnitte, um KI-gestützt weitere passende Inhalte zu finden. powered by