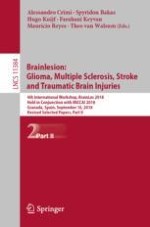
2019 | OriginalPaper | Buchkapitel
Segmentation of Brain Tumors and Patient Survival Prediction: Methods for the BraTS 2018 Challenge
verfasst von : Leon Weninger, Oliver Rippel, Simon Koppers, Dorit Merhof
Erschienen in: Brainlesion: Glioma, Multiple Sclerosis, Stroke and Traumatic Brain Injuries
Aktivieren Sie unsere intelligente Suche, um passende Fachinhalte oder Patente zu finden.
Wählen Sie Textabschnitte aus um mit Künstlicher Intelligenz passenden Patente zu finden. powered by
Markieren Sie Textabschnitte, um KI-gestützt weitere passende Inhalte zu finden. powered by