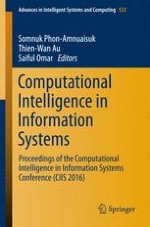
2017 | OriginalPaper | Buchkapitel
On Using Genetic Algorithm for Initialising Semi-supervised Fuzzy c-Means Clustering
verfasst von : Daphne Teck Ching Lai, Jonathan M. Garibaldi
Erschienen in: Computational Intelligence in Information Systems
Aktivieren Sie unsere intelligente Suche, um passende Fachinhalte oder Patente zu finden.
Wählen Sie Textabschnitte aus um mit Künstlicher Intelligenz passenden Patente zu finden. powered by
Markieren Sie Textabschnitte, um KI-gestützt weitere passende Inhalte zu finden. powered by