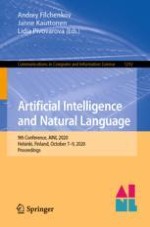
2020 | OriginalPaper | Buchkapitel
PolSentiLex: Sentiment Detection in Socio-Political Discussions on Russian Social Media
verfasst von : Olessia Koltsova, Svetlana Alexeeva, Sergei Pashakhin, Sergei Koltsov
Erschienen in: Artificial Intelligence and Natural Language
Aktivieren Sie unsere intelligente Suche, um passende Fachinhalte oder Patente zu finden.
Wählen Sie Textabschnitte aus um mit Künstlicher Intelligenz passenden Patente zu finden. powered by
Markieren Sie Textabschnitte, um KI-gestützt weitere passende Inhalte zu finden. powered by