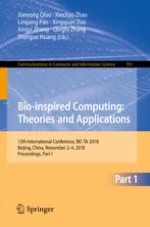
2018 | OriginalPaper | Buchkapitel
Research on Price Forecasting Method of China’s Carbon Trading Market Based on PSO-RBF Algorithm
verfasst von : Yuansheng Huang, Hui Liu
Erschienen in: Bio-inspired Computing: Theories and Applications
Verlag: Springer Singapore
Aktivieren Sie unsere intelligente Suche, um passende Fachinhalte oder Patente zu finden.
Wählen Sie Textabschnitte aus um mit Künstlicher Intelligenz passenden Patente zu finden. powered by
Markieren Sie Textabschnitte, um KI-gestützt weitere passende Inhalte zu finden. powered by