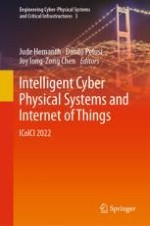
2023 | OriginalPaper | Buchkapitel
Term Frequency Tokenization for Fake News Detection
verfasst von : Pallavi Suresh, Abhishek Shettigar, M. Karunavathi, Ajith, M. G. Ramanath Kini
Erschienen in: Intelligent Cyber Physical Systems and Internet of Things
Aktivieren Sie unsere intelligente Suche, um passende Fachinhalte oder Patente zu finden.
Wählen Sie Textabschnitte aus um mit Künstlicher Intelligenz passenden Patente zu finden. powered by
Markieren Sie Textabschnitte, um KI-gestützt weitere passende Inhalte zu finden. powered by