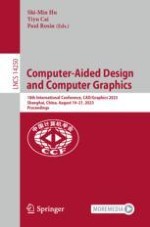
2024 | OriginalPaper | Buchkapitel
Unsupervised 3D Articulated Object Correspondences with Part Approximation and Shape Refinement
verfasst von : Junqi Diao, Haiyong Jiang, Feilong Yan, Yong Zhang, Jinhui Luan, Jun Xiao
Erschienen in: Computer-Aided Design and Computer Graphics
Verlag: Springer Nature Singapore
Aktivieren Sie unsere intelligente Suche, um passende Fachinhalte oder Patente zu finden.
Wählen Sie Textabschnitte aus um mit Künstlicher Intelligenz passenden Patente zu finden. powered by
Markieren Sie Textabschnitte, um KI-gestützt weitere passende Inhalte zu finden. powered by