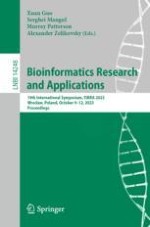
2023 | OriginalPaper | Buchkapitel
Unveiling the Robustness of Machine Learning Models in Classifying COVID-19 Spike Sequences
verfasst von : Sarwan Ali, Pin-Yu Chen, Murray Patterson
Erschienen in: Bioinformatics Research and Applications
Verlag: Springer Nature Singapore
Aktivieren Sie unsere intelligente Suche, um passende Fachinhalte oder Patente zu finden.
Wählen Sie Textabschnitte aus um mit Künstlicher Intelligenz passenden Patente zu finden. powered by
Markieren Sie Textabschnitte, um KI-gestützt weitere passende Inhalte zu finden. powered by