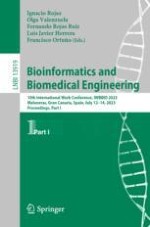
2023 | OriginalPaper | Buchkapitel
Recognition of Conformational States of a G Protein-Coupled Receptor from Molecular Dynamic Simulations Using Sampling Techniques
verfasst von : Mario Alberto Gutiérrez-Mondragón, Caroline König, Alfredo Vellido
Erschienen in: Bioinformatics and Biomedical Engineering
Verlag: Springer Nature Switzerland
Aktivieren Sie unsere intelligente Suche, um passende Fachinhalte oder Patente zu finden.
Wählen Sie Textabschnitte aus um mit Künstlicher Intelligenz passenden Patente zu finden. powered by
Markieren Sie Textabschnitte, um KI-gestützt weitere passende Inhalte zu finden. powered by