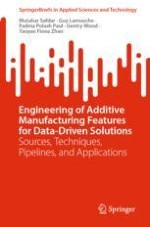
2023 | OriginalPaper | Buchkapitel
1. Introduction
verfasst von : Mutahar Safdar, Guy Lamouche, Padma Polash Paul, Gentry Wood, Yaoyao Fiona Zhao
Erschienen in: Engineering of Additive Manufacturing Features for Data-Driven Solutions
Verlag: Springer Nature Switzerland
Aktivieren Sie unsere intelligente Suche, um passende Fachinhalte oder Patente zu finden.
Wählen Sie Textabschnitte aus um mit Künstlicher Intelligenz passenden Patente zu finden. powered by
Markieren Sie Textabschnitte, um KI-gestützt weitere passende Inhalte zu finden. powered by